Statistics and Experimental Design: You’re Writing Your Hypothesis All Wrong
How to Avoid Common Pitfalls and Craft Testable, Data-Driven Hypotheses
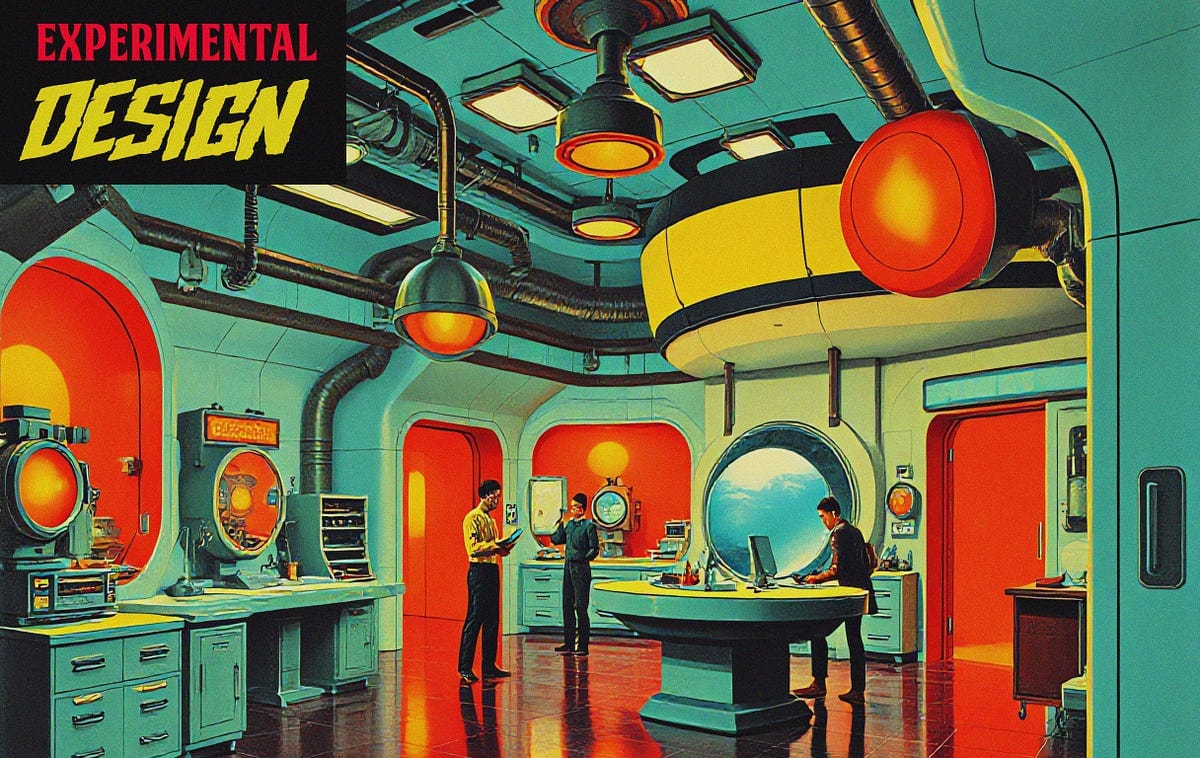
Every impactful statistical experiment starts with a question. Whether it’s a business leader asking, “What motivates customers to return after their first purchase?” or a data analyst investigating the impact of email marketing campaigns, research questions guide the journey toward answers. But how do you refine those broad questions into something actionable? And how do you structure studies to provide reliable insights? Let’s explore the art of experimental study statistics and the essential steps that make it work.
The Importance of a Good Research Question
Imagine starting a road trip without a destination in mind. That’s what research looks like without a clear question.
“The ability to ask the right question is more than half the battle of finding the answer.” Thomas J. Watson
A good research question serves as the compass for your experiment definition in statistics, ensuring every step aligns with your ultimate goal. To illustrate this, let’s evaluate an example marketing question using the three key qualities of specificity, alignment with goals, and feasibility.
For instance, consider the broad question: “How can we improve our email marketing strategy?”
- Step 1: Specificity: Broad questions like this are too vague to guide research effectively. Breaking it down leads to: “How does sending personalized email subject lines affect open rates?” By specifying the variable (personalized subject lines) and the outcome (open rates), the question becomes more focused and actionable.
- Step 2: Alignment with Goals: While improving email marketing is a general goal, aligning with the objective of increasing engagement further refines the question: “How does sending personalized email subject lines affect open rates over three months?” Adding a timeframe ties the question to measurable engagement goals.
- Step 3: Feasibility: A comprehensive strategy overhaul may exceed available resources. Refocusing the question to: “How does sending personalized email subject lines affect open rates over three months?” ensures it is achievable with existing tools and datasets, making it practical to implement.
Through these steps, the original vague question evolves into a robust research question that is clear, goal-aligned, and feasible.
By progressing through these steps, a vague and impractical question transforms into a clear, goal-aligned, and feasible statistical experiment example. This process ensures your study is both actionable and relevant.
“Statistics: The only science that enables different experts using the same figures to draw different conclusions.” — Evan Esar
Writing a Hypothesis Using the Email Marketing Example
Once you’ve nailed down your refined research question — “How does sending personalized email subject lines affect open rates over three months?” — the next step is crafting a hypothesis for your experimental statistics. A strong hypothesis serves as a blueprint for your study and addresses three essential components:
What Will Change? Identify the dependent variable — the outcome you’ll measure. In this case, the dependent variable is the open rate of email campaigns.
How Will It Change? Clearly state the expected direction of change. For example, you might hypothesize that open rates will increase with personalized subject lines.
What Will Cause the Change? Define the independent variable — the factor you’ll manipulate. Here, the independent variable is the use of personalized email subject lines.
Example Hypothesis: “If email campaigns include personalized subject lines, then open rates will increase over a three-month period compared to non-personalized campaigns.”
This hypothesis directly ties to your research question, is testable within a defined timeframe, and sets the foundation for an experimental study. Let me know if you’d like this integrated into the document!
“The true method of knowledge is experimentation.” — William Blake
Experimental vs. Observational Studies: Choosing the Right Path
“Experimentation is the mother of knowledge.” — Madeleine L’Engle
Before diving into data collection, decide whether your study will be experimental or observational:
- Observational Studies: These involve watching natural behaviors without interference. For example, tracking open rates for existing email campaigns without making any changes to subject lines.
- Experimental Studies: These introduce a change (or treatment) to measure its impact. For instance, testing whether personalized subject lines in email campaigns improve open rates over three months.
Each approach has its strengths. Observational studies provide valuable insights into current trends, while experimental studies allow you to test specific changes and measure their effects. The choice depends on your research goals and the level of control you require.
“Research is creating new knowledge.” — Neil Armstrong
The Steps of Experimental Design
Designing an experiment in statistics involves careful planning to ensure accuracy and reliability. Follow these steps:
- Formulate the Question: Be clear and specific. What exactly do you want to know?
- Craft the Hypothesis: Make it precise, testable, and rooted in existing knowledge.
- Identify Variables:
- Independent Variable: The factor you’ll change.
- Dependent Variable: The outcome you’ll measure. - Choose a Measurement Approach: Keep it simple. For example, an A/B test can compare two groups efficiently.
- Select an Analysis Method: Decide how you’ll interpret your results before starting. This ensures you collect the right data.
- Conduct the Study: Implement your plan, making adjustments as necessary.
Practical Examples of Experimental Study Statistics
Let’s bring these concepts to life with two scenarios:
- Marketing Example 1: Question: “Does sending emails with personalized subject lines increase open rates?” Hypothesis: “Emails with personalized subject lines will have higher open rates.” Experimental Design: Conduct an A/B test by sending one group emails with personalized subject lines and another group standard emails. Measure and compare open rates over a three-month period.
- Marketing Example 2: Question: “Does including customer testimonials in ads increase click-through rates?” Hypothesis: “Ads featuring customer testimonials will have higher click-through rates compared to those without testimonials.” Experimental Design: Run an A/B test comparing ads with and without testimonials, tracking click-through rates as the primary metric over a one-month campaign.
Why Experimental Design Matters
Great stats experiments don’t happen by chance. Experimental design ensures your study is structured, reliable, and focused on actionable insights. By asking the right questions, crafting strong hypotheses, and choosing the appropriate study type, you can uncover data-driven answers to complex challenges.
So, the next time you’re faced with a research problem, remember: It all starts with a question — and the design that follows determines the quality of your results.
References
Watson, T. J. (n.d.) Quoted in multiple sources.
Esar, E. (1968). 20,000 Quips & Quotes. Doubleday.
Blake, W. (n.d.). Quoted in multiple sources.
L’Engle, M. (n.d.). Quoted in multiple sources.
Armstrong, N. (n.d.). Quoted in multiple sources.